Introduction
In recent years, artificial intelligence (AI) has become a game-changing tool, altering many sectors of the economy and having far-reaching effects on our everyday lives. Knowledge of the artificial processes that make up AI is essential for realising its potential. This article will go into the essential components that give AI its “artificial” label and discuss the relevance of these components to the evolution of AI.
Recognising the “artificial” in AI
A. The First Steps in the Development of AI
The origins of artificial intelligence (AI) may be traced back to the middle of the twentieth century, when scientists first started to consider the potential of designing computers with human-level intellect. From primitive symbolic logic systems to recent advances in machine learning and deep learning, AI has come a long way.
B. Indicators of Artificiality in AI
1. An Attempt to Imitate Human Intelligence
The goal of artificial intelligence (AI) is, in part, to give robots human-level intellect. This necessitates simulating cognitive operations including perception, learning, thinking, and problem solving. Through modelling these facets of human intelligence, AI systems can handle challenging tasks and make sound judgements.
2. Processes of Machine Learning and Data Analysis
Machine learning is crucial to artificial intelligence because it allows computers to gradually become smarter by studying large amounts of data. Involves the use of models and algorithms to sift through large volumes of data in order to draw conclusions, automate processes, and optimise performance. For machine learning to work, data must be processed in some way, including collection, storage, pre-processing, and feature extraction.
3. Decision-Making and Automation
Decision-making and automation are two of AI’s most prominent artificial components. Through the use of intelligent devices, mundane operations may be streamlined, and efficiency can be maximised. In addition, AI systems use automated decision-making processes to evaluate data, spot trends, and choose optimal options. This allows AI to deal with difficult situations and help humans make decisions.
C. Importance of Synthetic Elements for AI Progress
To make progress in artificial intelligence, it is essential to comprehend the role that artificial components play inside systems. Researchers and developers can optimise AI algorithms, improve system performance, and handle ethical problems if they have a thorough understanding of how AI mimics human intellect, employs machine learning and data processing methods, and automates decision-making processes.
The Importance of Replicability in AI
An Analysis of Human Intelligence as a Foundation for Replication
Artificial intelligence (AI) systems owe a great deal to human intellect as a point of departure. Scientists’ goals in understanding human cognition include giving robots perception, learning, and problem-solving skills. To fully replicate human intellect, however, remains a formidable obstacle.
B. Replicating Human Thought
1. Perception and sensory information
By analysing various forms of sensory input, such as pictures, sounds, and texts, artificial intelligence systems attempt to mimic human experience. Applications such as picture recognition, audio recognition, and language translation are made possible through the use of computer vision and natural language processing algorithms to help computers read and understand these inputs.
2. Understanding and Logic
Machine learning techniques allow AI to learn from data and reason based on patterns and connections. Machines can learn, make predictions, and draw useful conclusions with the help of supervised, unsupervised, and reinforcement learning.
3. Problem-Solving and Imaginative Thinking
Artificial intelligence (AI) is programmed to solve difficult issues and display original thought. Algorithms like genetic algorithms and deep reinforcement learning allow computers to discover and optimise solutions, making them useful in fields as diverse as optimisation, gaming, and generative art.
C. Learning from Humans: Progress and Obstacles in Modelling Human Intelligence
Thanks to developments in machine learning, neural networks, and processing capacity, we have come a long way in our quest to give robots human-level intelligence. Problems still exist, though, and they include things like figuring out how the mind works, coping with ambiguity, and grasping the larger picture. Overcoming these limitations is essential for AI to reach its full potential.
The role of machine learning and data processing in AI
A. The Basics of Machine Learning
Machine learning is the use of algorithms and models to help AI systems learn from data and gradually enhance their functionality. These algorithms may be broken down into three distinct types:
1. Supervised learning
In supervised learning, artificial intelligence systems are taught using labelled data, in which both the input and the desired outcome values are specified. By analysing the tagged data for patterns and correlations, the algorithms can learn how to correctly translate input into output.
2. Unsupervised learning
Using unlabeled data, unsupervised learning teaches AI systems how to learn on their own. Without being given any labels beforehand, the algorithms are able to learn to recognise patterns, structures, and connections within the data. Cluster analysis, outlier identification, and exploratory data analysis are common applications of this method of learning.
3. Reinforcement learning
With reinforcement learning, an artificial intelligence agent learns to make judgements via a process of trial and error. The agent learns from the results of its actions in the form of reinforcement or criticism, and as a result, it improves its decision-making and performance over time. Games and robots both often use this strategy.
B. Information Processing in AI
Data processing is essential for AI systems since the quality and availability of data greatly affect their effectiveness. There are several steps in AI’s data processing pipeline:
1. Data collection
Artificial intelligence (AI) systems need massive amounts of data for training and improvement. Sensors, internet archives, user behaviours, and preexisting databases are just some of the data sources that may be mined. Data privacy and ethical concerns should be included in every step of the data gathering process.
2. Data storage and pre-processing
Once gathered, data must be stored in suitable forms and structures for effective processing. The purpose of pre-processing methods like data cleaning, normalisation, and transformation is to improve the quality of the data, get rid of any unwanted information or patterns, deal with missing numbers, and prepare the data for analysis.
3. Feature Extraction and Selection
Feature Extraction and Selection: Feature extraction is the process of identifying and removing noise from raw data to isolate useful information, or features. With this method, we want to record the most crucial features that aid the AI system in its learning and decision-making responsibilities. Feature selection methods aid in lowering dimensionality and computing complexity by determining the most informative and discriminative characteristics.
4. Data Cleaning and Quality Assurance
Errors, inconsistencies, and outliers in a dataset may be discovered and fixed during the data cleaning phase of quality assurance. That the data used for training and inference is trustworthy is guaranteed. Data validation and verification are two examples of quality assurance techniques used to identify and fix problems in the data processing pipeline.
Influence of Machine Learning and Data Processing on AI Skills
Advances in machine learning and data processing have allowed AI to make great strides in recent years. Artificial intelligence (AI) can currently do complicated tasks with great accuracy and efficiency by using massive volumes of data and complex algorithms. With the use of machine learning, AI systems may learn to see patterns, forecast outcomes, organise data, and adjust to new circumstances. An AI’s ability to train accurate models and draw trustworthy conclusions relies on its access to clean, relevant data, which can only be obtained via efficient data processing.
Thanks to these developments, AI is being used in many fields, such as language processing, computer vision, recommendation systems, autonomous cars, healthcare diagnostics, fraud detection, and economic forecasting, to name a few. However, issues like data privacy, bias in data and algorithms, and the interpretability of AI models need to be properly addressed to guarantee responsible and ethical AI research and implementation.
Decision-Making and Automation in Artificial Intelligence
A. The Importance of Robotics to Artificial Intelligence
Automation is an integral part of AI systems, allowing for the mechanisation of previously human-performed repetitive jobs. Business processes may be simplified, efficiency increased, and expenses decreased by using AI technology. Robotic process automation (RPA), chatbots, automated customer care, and industrial automation are just a few of the many areas where automation has been put to use.
1. Streamlining repetitive chores
AI-powered automation removes the need for people to undertake tedious and repetitive chores, freeing them up to concentrate on more sophisticated and strategic endeavours. Data input, document processing, inventory management, and routine customer inquiries are just a few examples of the kinds of jobs that may be performed effectively by AI systems, freeing up human resources for higher-value activities.
2. Optimising Efficiency and Productivity
Automation in AI enhances operational efficiency by decreasing error rates, shortening processing times, and maximising throughput. As a result of their ability to process massive amounts of data and complete jobs far more quickly than humans, AI systems have the potential to significantly boost efficiency and effectiveness in a variety of settings.
3. Reducing Human Errors
Errors made by humans should be minimised because of the financial and operational damage they may cause a company. The use of AI-powered automation reduces this danger since it gets rid of the room for human error. Artificial intelligence systems execute tasks in accordance with predetermined rules and algorithms, minimising the incidence of mistakes and the expenses that come with them.
B. AI system decision-making
1. Rule-Based Decision-Making
Automated decision-making according to predetermined rules and criteria may be a powerful tool in the field of artificial intelligence. By following these principles, AI systems may effectively react to a variety of situations and take the next logical step. Rule-based decision-making is widely utilised in fields including expert systems, robotic customer service, and fraud detection.
2. Statistical Analysis and Pattern Recognition
Artificial intelligence (AI) systems can sift through mountains of data in search of regularities and trends. Artificial intelligence is able to base its judgements on empirical evidence after it learns to identify patterns and connections within datasets. Predictive analytics, market research, credit scoring, and individualised suggestions are just some of the many applications for this method.
3. Reinforcement Learning for Decision-Making
Decision-Making Using Reinforcement Learning: Through the use of reinforcement learning methods, AI systems may discover the best methods for making decisions via trial and error. The AI agent is taught to execute behaviours that maximise rewards and minimise penalties by obtaining input in the form of incentives and punishments. This method shines in situations where there is a lot of uncertainty and movement, such as those seen in autonomous cars, robots, and AIs that play games.
C. Automated decision-making: moral and ethical considerations
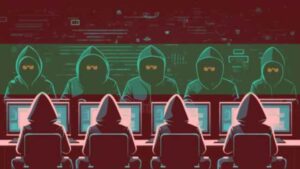
Even though there are many advantages to using automation and AI to make decisions, it is important to keep ethical concerns in mind. You should pay close attention to the following details:
1. Transparency and explainability
Because AI systems make judgements on their own, it’s crucial that they be transparent and able to provide an explanation for their reasoning. Users should be able to see the reasoning behind important choices, particularly in areas like healthcare, finance, and law. To better understand how AI systems arrive at their conclusions, researchers have developed interpretability algorithms and model explanation techniques.
2. Issues of fairness and bias
AI systems need to be built and educated to make decisions without favouritism or discrimination. Discriminatory results may be produced by biased training data or algorithms, which can then perpetuate existing social inequities. Steps should be taken to detect bias in data, algorithms, and decision-making processes and reduce its effects in order to promote justice and inclusion.
3. Supervision and Accountability
To guarantee the ethical use of artificial intelligence, it is important to have strict supervision and accountability mechanisms in place. Organisations need to set up systems for tracking, auditing, and fixing any ethical lapses or unintended effects caused by automated decision-making.
Addressing these ethical concerns will allow for the deployment of AI systems in a way that is consistent with social norms and that fosters trust and dependability.
Conclusion
In conclusion, the “artificial” part of artificial intelligence refers to the simulation of human intellect via machine learning, data processing, automation, and decision-making. Acquiring this knowledge is essential for maximising AI’s potential, expanding its capabilities, and dealing with its ethical consequences. We can have a significant and positive effect on the future of AI if we continue to expand our understanding in these areas.